Three lessons learned from the model that almost killed Wall Street!
By Sunny Zhang, Senior Solutions Architect, SAS
Back in 2000, David X. Li (then a quant at AXA Financial), developed an asset default correlation model using a simple and elegant approach. It was considered a breakthrough. Everyone was using it - from bond investors and Wall Street banks to ratings agencies and regulators. Traders and brokers were quoting prices for bonds based on correlations calculated by this model. Li’s model was so widely used that no one thought to ask about any associated risks.
Then the financial markets began to behave strangely – in ways that no one on Wall Street had expected.
This highly popular model could no longer measure the safety of securities. In other words, the increasing underlying defaults residing in the mortgage pools could not be reflected in the securities prices calculated from Li’s model. As a result, investors suffered huge losses – bringing down some of the nation’s largest institutions.
Model risk can never be eliminated, but it can be well-controlled.
Here are three things I believe contributed to the “failure” of Li’s model:
- The model assumptions. In Li’s model, the only factor that mattered to asset price was the single, aggregated default correlation number —one clean, simple, all-encompassing value that represented everything. It did not deal with the complex relationships of the underlying loans, which frankly can’t be summed in one correlation number. The model was an oversimplification of default correlation separated from the underlying loan defaults. In fact it was a ‘black-box’ – “very few people understood the essence of the model”, as Li himself said.
- The model development and validation methodology. The model was developed with limited CDS (Credit Default Swap) data history, which covered only a high performing period of time in the CDS market - when mortgage prices soared. The model was never validated with sufficient real world data that covered a broader range of conditions, not to mention including any adverse scenarios for stress testing. Therefore it simply left no room for unpredictability and variation in market conditions.
- The model misuse. Even the best model can be inappropriately applied to a scenario that it wasn’t designed for. Li's model was used to value hundreds of billions of dollars' worth of CDOs (Collateralized Debt Obligations) backed by risky mortgages. However, Li wrote the model for a ‘near perfect’ market state rather than for a real world filled with uncertainties. As such, it wasn’t suitable for factual risk management or valuation of derivatives. Recognizing the application misuse, Li said, "The most dangerous part is when people believe everything coming out of it.”
Ignoring these three elements puts any model at risk, which can result in significant financial losses, poor business decisions, or damage to a bank’s reputation.
What did we learn?
Due to the magnitude of the losses experienced during the last financial crisis, both FED and OCC regulators as well as those in other countries, have issued various regulations and guidance for model risk management. Banks now need to deal with increased scrutiny on the models that they heavily rely on and use for almost everything, from credit scoring, underwriting, pricing and collection to calculating capital and reserve adequacy for CCAR and Basel III.
Three key lessons learned:
- Know your models. Models can be a closed system, or "black box," with output that can be difficult to understand or interpret, particularly if the models were developed individually from different tools over different time periods. Rigor, due-diligence and transparency are required to understand how models are developed, the data used to validate them and what the model limitations are – like when or how they are to be used or not. To understand such questions, banks need to establish an integrated, robust model risk management strategy that is governed and traceable across all models. A single integrated platform enables this type of transparency.
- Stress test the model. Every model has its limitations. A popular or widely used model in the industry does not necessarily mean that model will also work well for your applications. Stress testing a model can spell out its limitations and ensures you know the scenarios in which the model does not function effectively. This type of rigor also helps forecast how the model could perform in future time horizons. For example, you can test and observe what inputs break the model, making it fail in its predictive power and as such, if that happens what other information is needed to supplement the model results. This would require a robust stress testing process and methodology.
- Challenge the model. Once developed, validated and deployed, models have to be constantly monitored and, as conditions change, updated – it’s an iterative process. According to the OCC Supervisory Guidance on Model risk Management released in April 2011, a central principle for managing model risk is the need for “effective challenge” of models. This means more than just establishing a Champion/Challenger routine to comparatively test, and if necessary, replace degrading models. More important is a well-structured model risk control function that oversees the entire model management lifecycle. This requires internal audit staff to verify that acceptable policies and model governance are in place and are followed. In fact, comprehensive documentation for this entire process makes model risk assessment and management more effective and promotes communication regarding compliance, policy, as well as recommendations, responses and exception tracking. A common risk management workflow and dashboard can facilitate this type of communication.
Model risk can never be eliminated but it can be well-controlled. SAS model risk management solutions and tools reside on a single, integrated model management framework that is open to scrutiny. With that, a bank will be able to effectively identify all sources of model risk and assess and control its magnitude in a documented, transparent and traceable workflow environment.
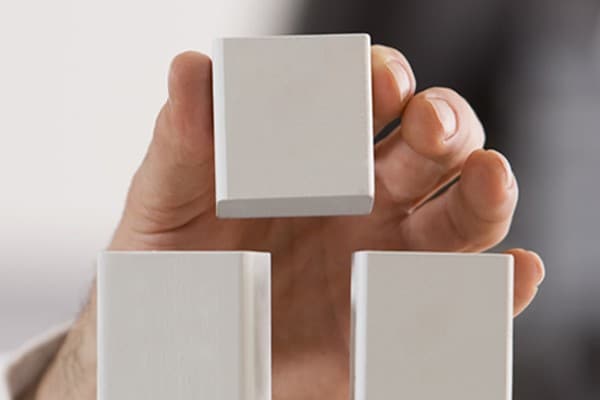
Read More
- What is a risk model? Your definition will affect your regulatory compliance path. Learn more in this article by Ravi Chari, a Risk Solution Architect at SAS.
- SAS has accelerated data mining model deployment throughout the analytical life cycle - read how in this paper: Time is precious, so are your models.