Analytic simulations: Using big data to protect the tiniest patients
By Daniel Teachey, SAS Insights Editor
A small, fragile life hangs in the balance. From hours-old premature newborns weighing less than a pound to months-old infants still struggling with medical conditions, neonatal intensive care units (NICUs) have to be ready for everything.
That’s why doctors, nurses and medical administrators in NICUs need every possible advantage to provide the best care possible to the most vulnerable patients.
But imagine having to make the decisions that affect your ability to actually provide care. How many beds do we need? How many nurses do we need? Which critically important quality improvement initiative do I tackle first? Where are our greatest opportunities to both improve outcomes and simultaneously reduce costs?
In the past, the primary way of answering these questions was through research, study and trial-and-error implementation. However, medical professionals like Dr. Chris DeRienzo, a neonatologist and Chief Quality Officer at Mission Health System, are looking at data on patients to provide a virtual way of testing different methods and approaches.
“We worked with SAS to create an analytics-based model of a NICU,” DeRienzo says. “The model actually creates simulated babies who work their way through a simulated NICU environment including simulated beds, which is even staffed by simulated nurses.”
The model actually creates simulated babies who work their way through a simulated NICU environment including simulated beds, which is even staffed by simulated nurses.
Dr. Chris DeRienzo
The model uses a vast resource of data to simulate the experience of patients, their conditions and staff responses in a computerized environment. In a study led by SAS and Duke University Health System and published in the Journal of Perinatology, the research applied a discrete event simulation model to predict outcomes and costs using pre-existing NICU data. DeRienzo first joined the research team during his neonatal fellowship at Duke, where his mentor, Dr. David Tanaka, was the impetus behind model’s development.
“We spent the first 18 months in data exploration trying to find the most significant characteristics to model,” DeRienzo says. “For example, we found that an infant’s gender was not a particularly significant factor in determining resource allocation. However, gestational age – meaning how many weeks a baby was able to spend inside his or her mother before being born prematurely – was (as expected) very significant.
“Next we evaluated the various complications that NICU babies can experience, like bloodstream infections, bleeding inside their brains and severe intestinal conditions. We then modeled the effect of these conditions both on a baby’s immediate acuity at the moment the complication happens as well as how each complication affects overall length of stay.” All of these attributes ultimately affect the global condition of each patient over time which, when combined across all patients, dictates the amount of resources a NICU needs to care for its population.
By simulating a variety of situations like this, DeRienzo and his colleagues could see how shifts in staffing affect a NICU’s broader ability to provide care. For example, when a patient experiences a critical complication, a nurse who normally covers three patients may have to focus on just that one, transitioning her two other patients to the care of other nurses. Modeling how frequently this happens in a virtual environment under multiple different scenarios can allow a NICU to optimize its staffing to be prepared for inevitable emergencies while maintaining day-to-day operations.
However, staffing isn’t the model’s only use. Since the simulation covers the entire environment, it can be used to ask and answer difficult questions about the relationships among things like financial metrics, operational metrics and clinical outcomes.
In their most recent publication, DeRienzo and his team showed that NICUs do not have a uniform correlation between length of stay and total cost of care. While it sounds odd, the simulation showed that a NICUs with the best published clinical outcomes (meaning, the fewest deaths and lowest rates of complications) actually had longer average stays than a NICU with the worst published clinical outcomes but markedly lower costs.
“In our model, the simulated NICU based on the best possible outcomes we could find published had a three days longer average length of stay compared to a unit with the poorest published outcomes,” DeRienzo says. “But interestingly, despite a longer average length of stay, the costs in the ‘best’ NICU were over $3 million less.
“This finding is critically important to health system leaders because it means a laser-like focus on length of stay as a uniform driver of resource utilization doesn’t paint the full picture. We must balance that focus with an equal emphasis on the clinical outcomes that drive total cost of care.”
Results like these will be critical to increasing the level of care for patients in the future.
“For us, it’s all about delivering better outcomes for our patients and their families, which then has a number of positive ripple effects,” DeRienzo says. “I think health care in 10 years will necessarily look very different from health care now, and we’re right in the middle of that transition. This project is just one example of how health care leaders can use innovative analytics tools to improve not only the ways we provide care but the actual care we provide.”
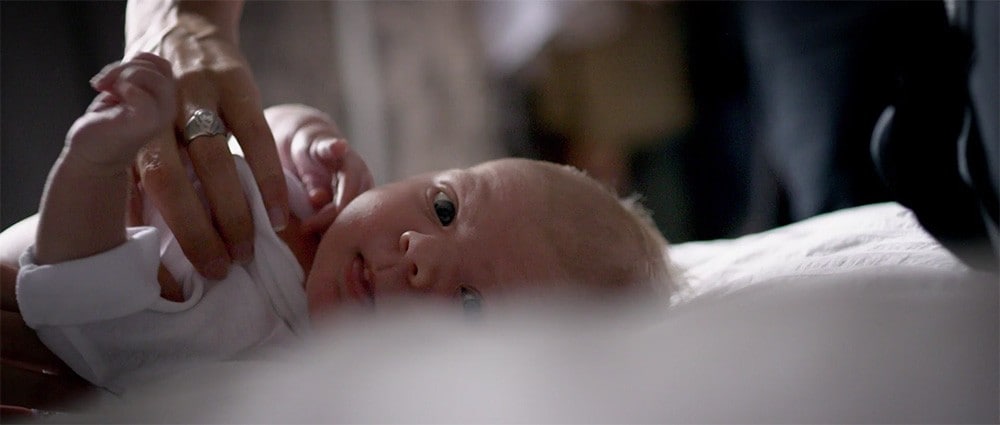
Get More SAS Insights
Want more Insights from SAS? Subscribe to our Insights newsletter. Or check back often to get more insights on the topics you care about, including analytics, big data, data management, marketing, risk and fraud.