Understand the propensity of your customers to defect
EDF Energy analyzes its customer base to build its marketing strategy
The UK energy market is characterized by high levels of churn. Since the market was completely opened up to competition in 1999, 19 million customers have changed suppliers, with many domestic consumers seeing savings of up to £150 on their energy bills. Currently, some 160,000 domestic consumers change electricity or gas supplier every week – an average switching rate of 38 percent.
The need to reduce churn is, therefore, a key operational focus for energy companies. With this in mind, EDF Energy's Customer Insight team uses SAS to deliver strategic analysis of data and gain new insight into the factors that motivate and drive customer behaviour. "We can meet customers' needs better by learning more about how they think, what they buy, what they use and how they want to interact with their supplier," says Clifford Budge, Customer Insight Manager, B2C Energy Sourcing and Customer Supply (ESCS), EDF Energy. SAS is helping the business to do precisely that.
It's all very well being able to manage two million records. It's when you have 400 different variables to test against that some solutions struggle – but SAS continues to perform well.
Clifford Budge
Customer Insight Manager
Managing churn using customer insight
EDF Energy has created a dedicated analytics function to focus on key areas including customer segmentation, churn assessment, probability modelling and product placement modelling. When the Customer Insight team was tasked with evaluating the technology marketplace and recommending a solution best suited to meeting its needs, including analysis on huge data volumes – data sets running into hundreds of millions of rows – its search led to SAS. Indeed, EDF Energy was already using SAS elsewhere in the organization and was happy with results being achieved.
The team's primary focus was churn modelling: evaluating the propensity for customers to leave the organization. Modelling involved examining a range of key metrics including 'life on supply' (older customers are likely to be more loyal than younger ones) and overall interaction levels. To deliver this functionality, EDF Energy implemented a SAS data management and predictive analytics platform. The first stage of the churn modelling process was to ensure the team has as much relevant data as possible. To support this, EDF Energy buys in third party data sets: attitudinal data to better understand customer attitudes, and lifestyle data with demographic details including people’s locations and household arrangements. Bringing these diverse data sources together is critical to optimize its modelling toolset.
Reducing losses: 'significant savings'
With SAS used to classify customer data and identify which customers are most likely to leave, EDF Energy then deploys a validation data set to monitor those customers. If they subsequently defect, the Customer Insight team takes the 'evidence' to the EDF marketing department and so help ensure it can prioritize customer communications based on identified risk. An additional focus is the company's 'dual fuel upgrade to reduce churn' program. "The aim is to understand which of our customers is currently 'electricity only' but are also mains gas capable: they can take dual fuel," explains Budge.
EDF Energy built SAS models that predicted the top 25 percent of customers covered are four times more likely to take dual fuel - and are therefore far less likely to churn. Equally, by identifying those customers least likely to take action, EDF Energy can save money that might otherwise have been wasted trying to sell upgrades to them. "If you were a supplier that suffered one million customer losses every year, with the top 25 percent of customers billing an average of £1,200 per year, that equates to a total risk from those customers alone of around £300 million a year," Budge adds. "Even if you can action just 5 to 10 percent of that, you are talking about a significant savings."
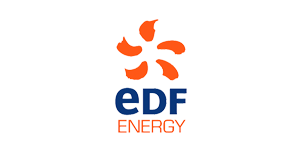
Challenge
Identifying and reducing churn levels in a highly competitive market: the average switching rate is 38%.
Solution
A SAS data access, data management and predictive analytics platform focused on churn and propensity modeling.
Benefits
- The ability to quickly, easily and accurately evaluate large volumes of data.
- Test against multiple variables.
- Incorporate a broad range of modelling techniques including logistic regression.
- Deliver new customer insight to help reduce churn.
Bu makalede gösterilen sonuçlar, burada açıklanan belirli durumlara, iş modellerine, veri girdilerine ve hesaplama ortamlarına özeldir. Ticari ve teknik değişkenlere bağlı olarak, her bir SAS müşterisinin deneyimi kendine özgüdür ve hiçbir açıklama tipik olarak düşünülmemelidir. Gerçekleşen tasarruflar, sonuçlar ve performans özellikleri, bireysel müşteri konfigürasyonlarına ve koşullara göre değişiklik gösterecektir. SAS, her müşterinin benzer sonuçlara ulaşacağını garanti etmemekte veya bu konuda bir taahhütte bulunmamaktadır. SAS ürün ve hizmetlerine yönelik tek garanti, söz konusu ürün ve hizmetlere dair yazılı anlaşmada belirtilen açık garanti beyanlarında ifade edilen garantilerdir. Bu belgedeki hiçbir ifade, ek bir garanti teşkil ettiği yönünde yorumlanmayacaktır. Müşteriler, üzerinde anlaşılmış bir sözleşmeden doğan bir alış verişin bir parçası olarak ya da SAS yazılımının başarılı bir şekilde uygulanmasının ardından sunulan proje başarı özetinde, başarılarını SAS ile paylaşmışlardır. Marka ve ürün adları, söz konusu şirketlerin ticari markalarıdır.