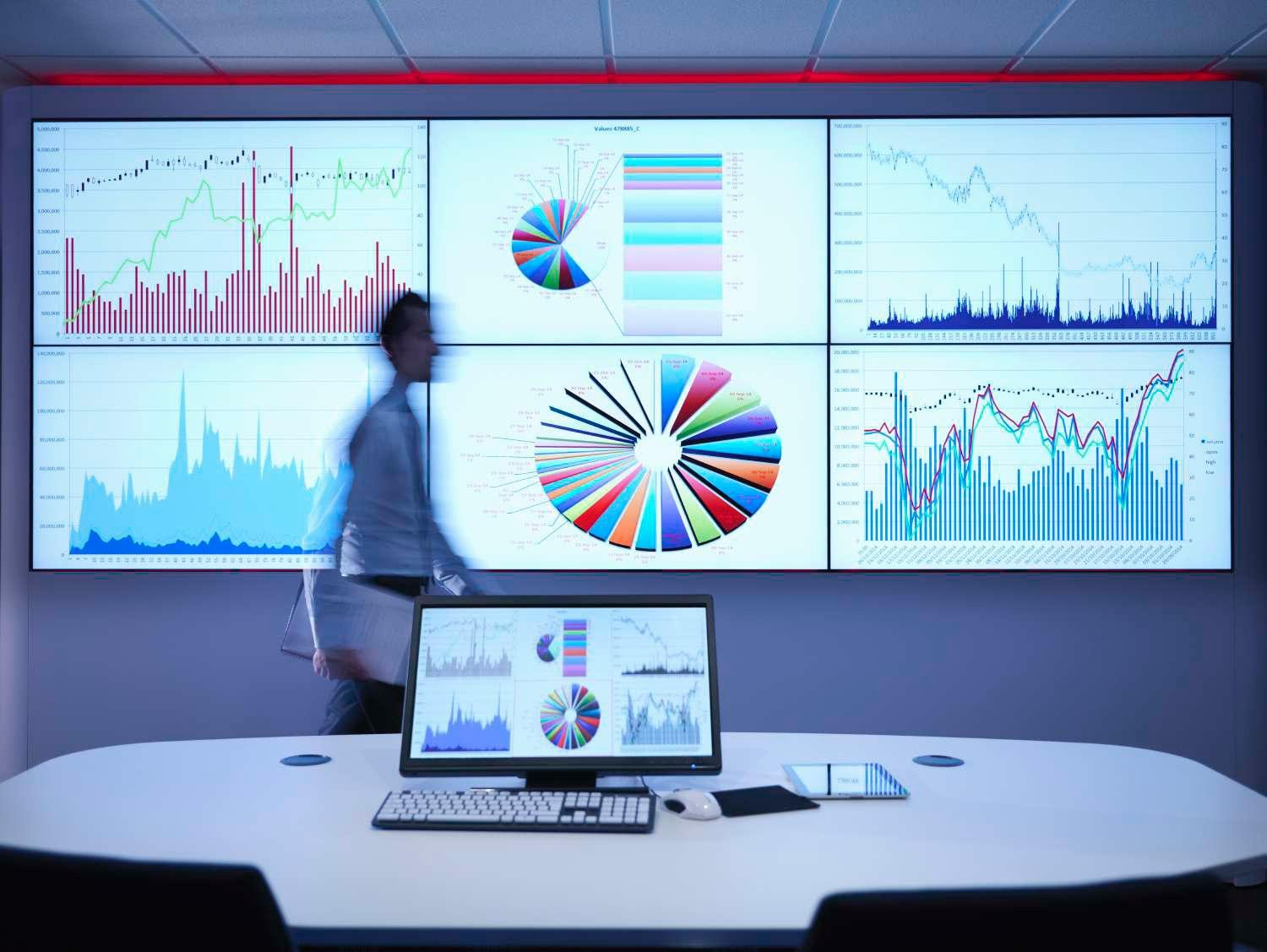
Key questions to kick off your data analytics projects
By: Phil Simon, author, speaker and noted technology expert
I’ve been around data my entire professional life. All my books deal with data in one way or another. I worked as an enterprise-systems consultant for a decade. And then there’s academia. I teach the analytics capstone course at Arizona State University, and I’ve overseen more than 50 data analytics projects for groups and nearly 200 individual ones. By now, I like to think I know a thing or six about the practice of analytics.
Here’s perhaps the most crucial: There’s no single blueprint for beginning a data analytics project – never mind ensuring a successful one.
However, I have found that the following questions help individuals and organizations frame their data analytics projects in instructive ways. Put differently, think of these questions as more of a guide than a comprehensive how-to list.
Oddly enough, I struggle with the very idea of an analytics project. I’ve even argued against the approach in the past because it’s more important to imbue analytics into a culture than do one-off projects. For the purposes of this article, however, I’ll put aside semantic quibbling and jump right in.
Is this your organization’s first attempt at a data analytics project?
When it comes to data analytics projects, culture matters.
Consider Netflix, Google and Amazon. All things being equal, organizations like these have successfully completed data analytics projects. Even better, they have built analytics into their cultures and become data-driven businesses. As a result, they will do better than neophytes. Fortunately, first-timers are not destined for failure. They should just temper their expectations.
Data, Analytics and AI: How Trust Delivers Value
Read the results of the annual data and analytics global executive study with MIT Sloan Management Review – based on a survey of 2,400 business leaders and managers.
What business problem do you think you’re trying to solve?
This might seem obvious, but plenty of folks fail to ask it before jumping in. Note here how I qualified the first question with “do you think?”. Sometimes the root cause of a problem isn’t what we believe it to be; in other words, it's often not what we at first think.
In any case, you don’t need to solve the entire problem all at once by trying to boil the ocean. In fact, you shouldn’t take this approach. Project methodologies (like agile) allow organizations to take an iterative approach and embrace the power of small batches.
What types and sources of data are available to you?
To be sure, most if not all organizations store vast amounts of enterprise data. Looking at internal databases and data sources makes sense. Don’t make the mistake of believing, though, that the discussion ends there.
External data sources in the form of open data sets (such as data.gov) continue to proliferate. There are easy methods for retrieving data from the web and getting it back in a usable format – scraping, for example. This tactic can work well in academic environments, but scraping could be a sign of data immaturity for businesses. It’s always best to get your hands on the original data source when possible.
Caveat: Just because the organization stores it doesn’t mean you’ll be able to easily access it. Pernicious internal politics stifle many an analytics endeavor.
What types and sources of data are you allowed to use?
With all the hubbub over privacy and security these days, foolish is the soul who fails to ask this question. As some retail executives have learned in recent years, a company can abide by the law completely and still make people feel decidedly icky about the privacy of their purchases. Or, consider a health care organization – it may not technically violate the Health Insurance Portability and Accountability Act of 1996 (HIPAA), yet it could still raise privacy concerns. Another example is the GDPR. Adhering to this regulation means that organizations won’t necessarily be able to use personal data they previously could use – at least not in the same way.
What is the quality of your organization’s data?
Common mistakes here include assuming your data is complete, accurate and unique (read: nonduplicate). During my consulting career, I could count on one hand the number of times a client handed me a “perfect” data set. While it’s important to cleanse your data, you don’t need pristine data just to get started. As Voltaire said, “Perfect is the enemy of good.”
What tools are available to extract, clean, analyze and present the data?
This is 2018, not 1998. Please don’t tell me that your analytics efforts are limited to spreadsheets.
Sure, Microsoft Excel works with structured data – if the data set isn’t all that big. Make no mistake, though: Everyone’s favorite spreadsheet program suffers from plenty of limitations, in areas like:
- Handling semistructured and unstructured data.
- Tracking changes/version control.
- Dealing with size restrictions.
- Ensuring governance.
- Providing security.
For now, suffice it to say that if you’re trying to analyze large, complex data sets, there are many tools well worth exploring. The same holds true for visualization. Never before have we seen such an array of powerful, affordable and user-friendly tools designed to present data in interesting ways. For instance, SAS® Visual Analytics, SAS® for Machine Learning and Deep Learning and several open source tools are just some applications and frameworks that make data visualization powerful and, dare I say, cool.
Caveat 1: While software vendors often ape each other’s features, don’t assume that each application can do everything that the others can.
Caveat 2: With open source software, remember that “free” software could be compared to a “free” puppy. To be direct: Even with open source software, expect to spend some time and effort on training and education.
What will an individual, group, department or organization do with keen new insights from your data analytics projects? Will the result be real action? Or will a report just sit in someone’s inbox? Phil Simon Author, speaker and technology expert
Do your employees possess the right skills to work on the data analytics project?
The database administrator may well be a whiz at SQL. That doesn't mean, though, that she can easily analyze gigabytes of unstructured data. Many of my students need to learn new programs over the course of the semester, and the same holds true for employees. In fact, organizations often find that they need to:
- Provide training for existing employees.
- Hire new employees.
- Contract consultants.
- Post the project on sites such as Kaggle.
- All the above.
Don't assume that your employees can pick up new applications and frameworks 15 minutes at a time every other week. They can’t.
What will be done with the results of your analysis?
In Analytics: The Agile Way, I penned a case study about how one company’s recruiting head honcho asked me to analyze applicant data in 1999. The company routinely spent millions of dollars recruiting MBAs at Ivy League schools only to see them leave within two years. Rutgers MBAs, for their part, stayed much longer and performed much better.
Despite my findings, the company continued to press on. It refused to stop going to Harvard, Cornell, etc. because of vanity. In his own words, the head of recruiting just “liked” going to these schools, data be damned.
Food for thought: What will an individual, group, department or organization do with keen new insights from your data analytics projects? Will the result be real action? Or will a report just sit in someone’s inbox?
What types of resistance can you expect?
You might think that people always and willingly embrace the results of data-oriented analysis. And you’d be spectacularly wrong.
Case in point: Major League Baseball (MLB) umpires get close ball and strike calls wrong more often than you’d think.1 Why wouldn’t they want to improve their performance when presented with objective data? It turns out that many don’t. In some cases, human nature makes people want to reject data and analytics that contrast with their world views. Years ago, before the subscription model became wildly popular, some Blockbuster executives didn’t want to believe that more convenient ways to watch movies existed.
Caveat: Ignore the power of internal resistance at your own peril.
What are the costs of inaction?
Sure, this is a high-level query and the answers depend on myriad factors. For instance, a pharma company with years of patent protection will respond differently than a startup with a novel idea and competitors nipping at its heels. Interesting subquestions here include:
- Do the data analytics projects merely confirm what we already know?
- Do the numbers show anything conclusive?
- Could we be capturing false positives and false negatives?
Think about these questions before undertaking data analytics projects
Don’t take the queries above as gospel. By and large, though, experience proves that asking these questions frames the problem well and sets the organization up for success – or at least minimizes the chance of a disaster.
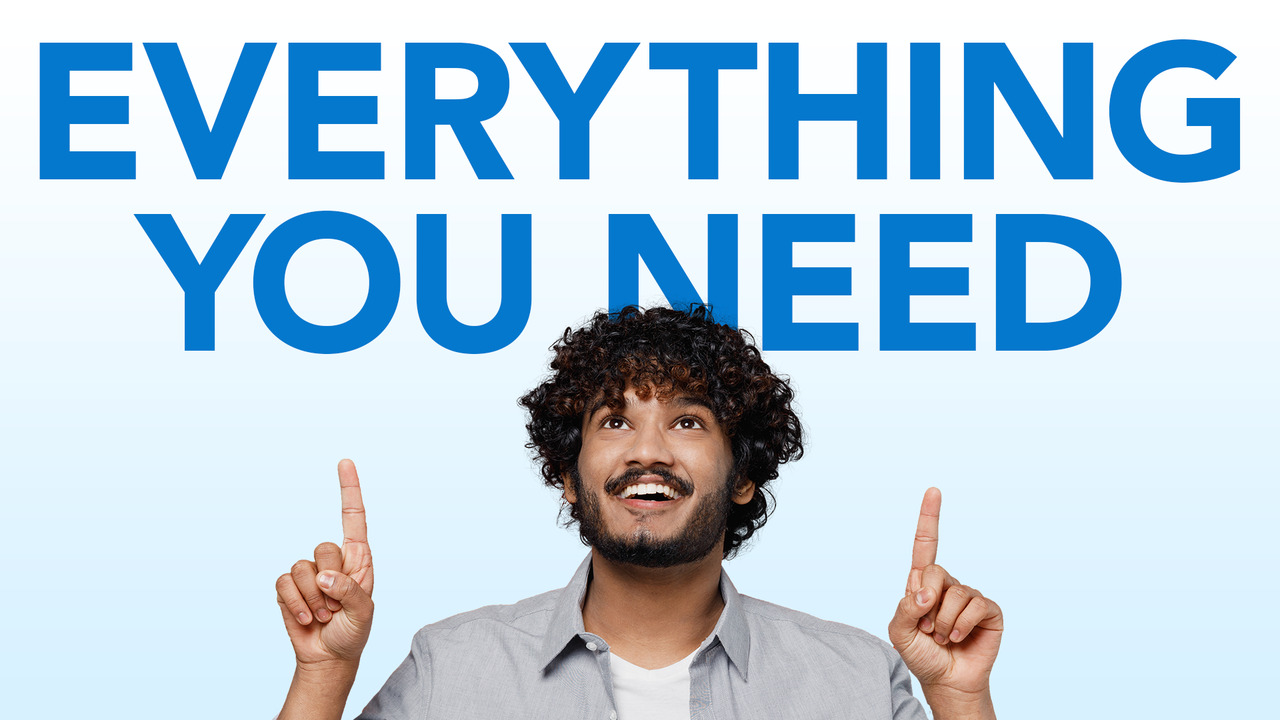
- 2x
- 1.75x
- 1.5x
- 1.25x
- 1x, selected
- 0.75x
- 0.5x
- Chapters
- descriptions off, selected
- subtitles settings, opens subtitles settings dialog
- subtitles off, selected
This is a modal window.
Beginning of dialog window. Escape will cancel and close the window.
End of dialog window.
This is a modal window. This modal can be closed by pressing the Escape key or activating the close button.
This is a modal window. This modal can be closed by pressing the Escape key or activating the close button.
This is a modal window. This modal can be closed by pressing the Escape key or activating the close button.
This is a modal window. This modal can be closed by pressing the Escape key or activating the close button.
About the author Phil Simon is a keynote speaker and recognized technology expert. He is the award-winning author of eight management books, most recently Analytics: The Agile Way. He consults organizations on matters related to strategy, data, analytics and technology. His contributions have been featured on The Harvard Business Review, CNN, Wired, The New York Times, and many other sites. In the fall of 2016, he joined the faculty at Arizona State University’s W.P. Carey School of Business (Department of Information Systems).
References
1.Umps get 1 in 3 close pitches wrong, HBO story shows.
Recommended reading
-
4 strategies that will change your approach to fraud detectionAs fraudulent activity grows and fighting fraud becomes more costly, financial institutions are turning to anti-fraud technology to build better arsenals for fraud detection. Discover four ways to improve your organization's risk posture.
-
Detect and prevent banking application fraudCredit fraud often starts with a falsified application. That’s why it’s important to use analytics starting at the entrance point. Learn how analytics and machine learning can detect fraud at the point of application by recognizing the biggest challenge – synthetic identities.
-
Online payment fraud stops hereBillions of dollars each year are lost to online payment fraud through channels that provide convenient – yet vulnerable – ways to shop and bank. See how to fight back and win with advanced analytics.