Are you covering who you think you’re covering?
By Julie Malida, Principal, Health Care Fraud Solutions, SAS
In my last post, I talked about payment integrity and how health care organizations (HCO) are implementing it as a strategy for containing costs. There are many aspects to payment integrity, which covers many types of improper billings. One important aspect is eligibility fraud.
How rigorous are you in determining membership eligibility? If you are not diligent enough, you may be letting money slip out the door. In fact, by some estimates, between 4 and 18 percent of all health plan benefits are paid out in error due to eligibility fraud issues. Examples include:
- A member may fail to fully report income.
- A member’s status might change sometime after he applies for coverage, and he fails to report it.
- Perhaps a person’s student status changes.
- A spouse may have gained other coverage, or the person divorced and neglected to tell you about it.
- Or maybe that person was never legally married to begin with.
Other forms of eligibility fraud include member ID card sharing, card theft or collusion between individuals or with providers.
Advanced analytic solutions when applied to a broad range of data can help you identify eligibility fraud. Analytics can churn through the data quickly, search for patterns and flag anything that looks suspicious. The broader the range of data types you use, the more likely you are to spot inconsistencies and anomalies that could indicate eligibility fraud.
If you are not already using these, here are other data sources to consider in flagging potential eligibility fraud:
- By examining past claims data, you can build a vector of an individual’s use pattern. You can also learn what benefits the person had in the past. Someone who’s had an appendectomy, will not need another in the future. Therefore, it might not be the same person trying to claim benefits.
- Electronic medical records can supplement existing member information. As with claims data, a sudden change in pattern may indicate a member ID card was shared or stolen.
- Watch lists (such as those from the National Health Care Anti-Fraud Association, the National Insurance Crime Bureau and the US Department of Health and Human Services’ List of Excluded Individuals/Entities) can tip you off to existing fraudsters.
- Call center logs can be a surprising source of insight. You can use text mining tools to extract information from these logs and add it to existing data on providers and members.
- Government programs, such as those for SNAP and energy assistance, along with child support and adoption information, can also be used to validate eligibility and monitor usage.
- Corporate information from trusted sources, such as Dun & Bradstreet and InfoUSA can yield clues to an individual’s assets.
- Property tax records are another good source of information for verifying assets. But due to data transformation and extraction issues (counties store their information differently), these may be more useful for supporting investigations than for initially flagging suspicious entities.
- Employment records are useful for verifying income levels and hours worked.
- The Social Security death master file and state vital records can help identify illogical or impossible claims. You may find someone who passed away in one state and suddenly popped up as eligible for benefits in another.
- Public and purchased search databases, such as Experian and TransUnion credit reports, can provide detailed information about individuals. These databases might even reveal past fraudulent behavior or financial difficulties that might make someone more apt to try to game the system.
- Address verification data from the US Postal Service can confirm whether a given address is correct or if it even exists – and isn’t a drop box or a vacant lot in some industrial zone.
Your data on previously detected eligibility fraud, waste and abuse can also help you build your predictive models. An analytics solution can then identify and flag other individuals who are beginning to exhibit the same behaviors or patterns.
Incorporating multiple, diverse data sources into your analysis allows you to create a far more comprehensive picture of your member base. It also brings new insight for spotting eligibility fraud.
Read the full white paper: Before Claims Fraud, What About Eligibility Fraud?
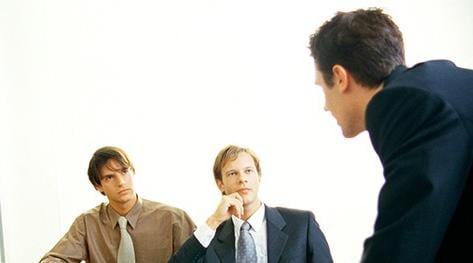
Read More
Read the full white paper: Before claims fraud, what about eligibility fraud?