Analytics for all, all in one place
Model governance with an analytics platform supports best-of-breed analytics
By Alison Bolen, SAS Insights Editor
Let me introduce you to some smart, analytically minded employees at a growing national bank who are each seeing positive results with analytics in their own divisions:
- James is a data scientist in the business development group who analyzes data to create customized offers for the bank’s premium customers.
- Susan is a digital marketer who tracks and influences the customer journey for prospective mortgage customers.
- Christina is a risk analyst who builds risk models for the bank’s loan portfolios.
- Marvin is a citizen data scientist in a top retail banking location who takes it upon himself to analyze data about local customers at his branch.
Between them, these enterprising employees are using a dozen different packages for analytics and data management. From Informatica and SAS to Python and R, some of these applications are open source, some are cloud-based and some are hosted as enterprise applications.
How can the IT department at this bank make sure all of these projects are using trustworthy data, accurate models and a rigorous process of analysis that will guarantee useful results? And whose responsibility is it to stitch all of these disparate code bases and business scenarios together to trace the customer journey or find other opportunities for analytics?
A modern analytics platform handles the diversity of analytics challenges.
A single ecosystem that supports model governance
What this bank needs is one place to combine data for analysis, consolidate all of its analytics endeavors for tracking and monitoring, and provide appropriate access to models across divisions. But how can one platform corral all that analytics activity, provide easy access for all levels of users, and provide model governance across all types of analytics projects?
Sarah Gates, Analytics Platform Marketing Manager as SAS, recommends a centralized analytics platform that is inclusive, scalable and resilient. “A unified platform that works with your IT requirements to govern the entire analytics lifecycle gives you the ability to quickly adapt to the full spectrum of analytic opportunities in your organization, foster innovation and streamline deployment of the results."
The platform should be accessible to different types of skills sets, including providing data management capabilities, analytics functionality and model governance for data scientists.
“It needs to govern all analytics, not just some,” says Gates, especially open source packages that don’t come with their own governance functionality.
“You need an analytics platform that unifies and governs your complex and diverse analytic ecosystem.” says Gates. “That is key for driving business value from your data while mitigating risk.”
Free white paper
Operationalizing and Embedding Analytics for Action: A TDWI Best Practices Report describes current strategies and future trends for embedded analytics across both organizational and technical dimensions, including organizational culture, infrastructure, data and processes.
Download the report
Model governance through an analytics platform
So what is an analytics platform? It’s software that allows you to connect all the parts of your analytics ecosystem. It works behind the scenes to help data scientists build and manage models. It helps the business quickly get value from analytics. It helps IT from a governance and compliance perspective by providing information about model versioning, audit trails, model lineage and source data.
And how does an analytics platform support model governance? It provides data scientists with a single platform to leverage throughout the entire analytics lifecycle. The platform provides them with a centralized repository of data, an inventory of analytic methods and a standardized code base that can be incorporated into any deployment activity or action. They are able to build, compare, and select models regardless of the language in which they were created. Model results are easy to interpret, ensuring they are meeting the business need. Code is portable, so it can be defined once, run anywhere and scaled to solve any size problem. Finally, they can register models for deployment. Business analysts apply business rules to describe the conditions in which a model should trigger. Everything is documented and tested for deployment purposes.
As a result, IT understands the business context associated with every model developed. Models don’t have to be recoded into another language before deployment. In all these ways the platform ensures effective model governance as well as accurate results through monitoring of model inventory, performance and deployment.
“It’s not unusual for data scientists to recode models in a different language and wonder why the results don’t match,” says Oliver Schabenberger, Executive Vice President, Chief Operating Officer and Chief Technology Officer at SAS. “With an analytics platform, you can request analytics on the same data from different languages, and you don’t have to worry about idiosyncrasies and differences of algorithm implementation, options and data models. Consistency and standardization on the back end remove worries about differences on the front end.”
An open, inclusive analytics platform allows all data users to stand behind the best algorithm. It doesn’t matter what language you write it in, or where you drive it from – you know it will be accurate.
“With a platform in place, it’s no longer an argument between an algorithm in an R package or a Python library,” says Schabenberger. “The languages execute the same analytic code on the same data model, no matter how you trigger it. And all languages reap the benefits of multithreading, distributed computing, common data access and security models.”
Schabenberger compares the model governance capabilities of an analytics platform to web governance, explaining that you can pull up a webpage from multiple different laptops, tablets or smartphones and still see the same content. Web governance standards allow that to happen.
Analytics model governance works the same way. You can pull in data and build models from different systems throughout the organization, and the analytics platform ensures consistency, accuracy and performance.
Open and enterprise analytics together in one platform
Let’s get back to the bank from the beginning of this article. An analytics platform lets this organization be open enough that James, Susan, Christina and Marvin can all use their preferred tools, and the bank can guarantee consistent enterprise-class results across all the different packages. IT now governs analytics implementation and the data to make sure everyone is working against the same data model. It also ensures IT is able to govern the use of data to comply with regulatory requirements.
When so many different users are accessing data in different ways, an analytics platform is the foundation for becoming a data driven organization. "An analytics platform positions you to deliver business value from analytics, from concept to innovation and implementation to ROI,” says Gates.
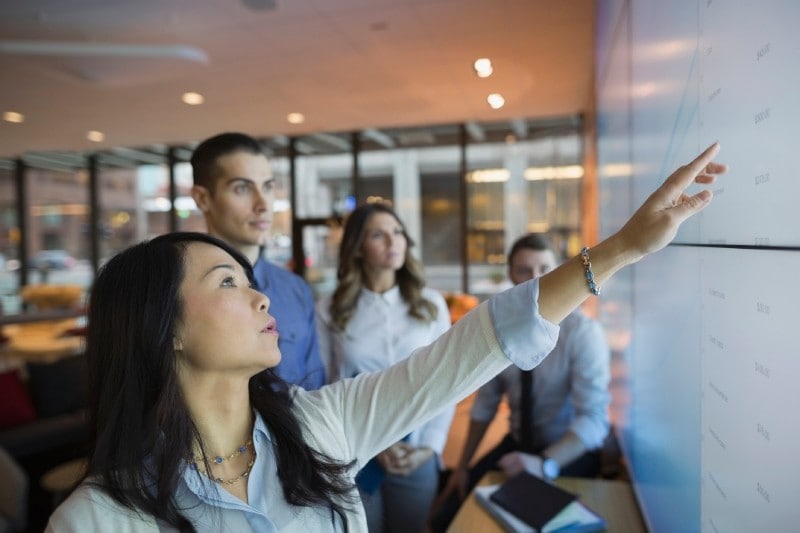
Read Next
- Improve your analytics governance with SAS Viya®
- Are there benefits in using both commercial and open source analytics? Find out in the infographic, A Powerful Pair.
If you waited 3 months for analytics results you could have had in 3 clicks ... let's change that.
Get More Insights
Get More SAS Insights
Want more Insights from SAS? Subscribe to our Insights newsletter. Or check back often to get more insights on the topics you care about, including analytics, big data, data management, marketing, risk and fraud.