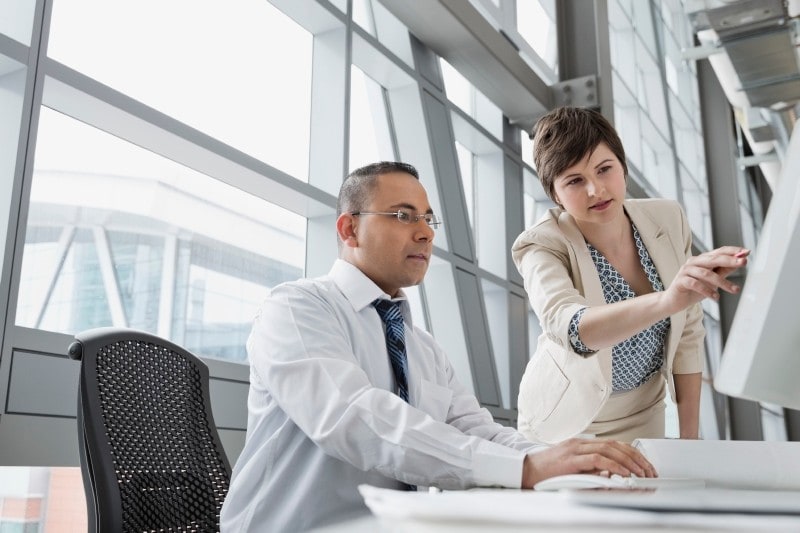
Operationalizing analytics: 4 ways banks are conquering the infamous ‘last mile’
By Anne-Lindsay Beall, SAS Insights Editor
The banking industry is further along in analytics maturity than most, depending on analytical modelling to assess credit and portfolio risk, comply with government regulations, determine what financial products to offer and so much more. And yet banks are challenged to realize the full benefits of their modeling investment, especially when it comes down to the “last mile” of analytics – the gap between great analytic output and actually deriving value from it.
The number one reason for missing out on their new models' potential? The lack of a repeatable process for operationalizing analytics that quickly moves models into production and monitors performance over time. According to Gartner, less than 50% of the best models get deployed, and 90% of models take more than three months to deploy. That leaves analysts frustrated, valuable data wasted and big strategic decisions in question.
The solution is a unified analytics strategy that embeds analytics in all areas of the organization to drive decision making. Here are four examples across the banking industry that show how these leading organizations followed a clearly defined path to put analytics in action to solve specific business challenges -- and the results they achieved.
Get the most from your AI investment by operationalizing analytics
Download this free white paper to learn how ModelOps ensures maximum business impact from analytics, automates repeatable tasks, builds collaboration between stakeholders and streamlines the flow of analytics into decision making processes.
1. Challenge: IFRS 9 compliance
The International Financial Reporting Standard 9 (IFRS 9) created additional work and complexity for banks, requiring provisioning models that are unbiased and forward looking for all financial assets that are not fair valued through profit and loss.
One of the largest multinational banks in the world came to SAS to develop a comprehensive strategic solution to build, deploy and manage more than 200 models to comply with IFRS 9 regulations, and put reporting mechanisms in place to handle huge amounts of data from across its global operations.
What they did:
The bank began implementing an analytics platform from SAS to support deployment of models that were being built in parallel.
While deployment of SAS and launch of the bank’s IFRS 9 program were complex, requiring source data from 60 to 70 systems and models covering all of the bank’s financial assets, within two months the bank had already begun implementing models and components on the SAS platform.
Within three months, the bank had put in place an agile implementation process for delivery of new models every two weeks with SAS providing support in configuration.
Results:
- The bank gained a centralized, flexible and high-performance environment to meet the challenges of expected loss modeling — while advancing risk management and financial reporting.
- 17% more efficient model deployment in support of IFRS 9.
- 60% more efficient model maintenance and management.
Less than 50% of the best models get deployed, and 90% of models take more than three months to deploy. That leaves analysts frustrated, valuable data wasted and big strategic decisions in question.
2. Challenge: Digital transformation
Like many banks, this Canadian financial institution wanted to transform into a digital organization – to not only build new channels to reach customers, but to also communicate with customers in their preferred channel. The problem? Disparate analytic tools and a fragmented analytic landscape.
What they did:
They partnered with SAS to create a unified and agile end-to-end data science platform deployed in the cloud so that new capabilities could easily be added. By moving to a data lake, the organization minimized costly and inefficient data movement and replication.
Results:
- Now powered with a modernized and agile SAS Viya infrastructure in the Google Cloud, this financial institution can quickly model and analyze big data to improve the customer experience and produce business value.
- With in-memory and in-database processing, they’ve cut processing time in half.
- All users, regardless of coding language preference, can visualize analytics.
- The bank has transformed into a digital enterprise that provides a top-notch, consistent customer experience across channels.
3. Challenge: Accurate risk scoring and analysis
Three regional banks in Europe were in danger of being downgraded by regulators and rating companies because they lacked a sound credit risk management process. They each struggled with lengthy time-to-market when changing scoring models. The analytical life cycle process for credit score models took more than a year — impacting risk in the portfolio. In addition, data gathering, analytics and reporting processes were very manual, reducing each banks’ control of portfolios.
What they did:
The banks implemented SAS solutions to speed time-to-market for scoring models, create a sound credit risk management process and trace changes in that process.
Results:
- Prebuilt data management processes helped the banks achieve a shorter time to market for credit risk models and more accurate risk scores for customers.
- Improved credit risk management, more accurate risk scores for customers, and flexible pricing that improved the banks’ ability to introduce new products quickly.
- Increased accuracy and consistency of credit risk analytics — using enhanced data — benefited each bank’s bottom line.
- Dramatically reduced the on-boarding time of new analytics use cases.
- Saved over $125 million by consolidating the IT infrastructure to a new open source environment.
- Improve analysts' efficiency by 30-40%.
- Helped influence net new revenues of $1 billion.
Conquering the 'last mile'
The last mile is always the hardest, but these banks succeeded where many fail because they implemented structured processes to coordinate resources across analytics, IT and the business. They quickly deployed the best solutions, got models into production quickly, and integrated analytics throughout the business, helping the entire organization make better decisions faster. And if they did it – you can too.
Recommended reading
-
6 ways big data analytics can improve insurance claims data processingWhy make analytics a part of your insurance claims data processing? Because adding analytics to the claims life cycle can deliver a measurable ROI.
-
Modern manufacturing's triple play: Digital twins, analytics & IoT IoT-powered digital twins revolutionize manufacturing with real-time data analysis, predictive maintenance and optimized production. Discover their transformational impact.
-
Key questions to kick off your data analytics projectsThere’s no single blueprint for starting a data analytics project. Technology expert Phil Simon suggests these 10 questions as a guide.
Ready to subscribe to Insights now?