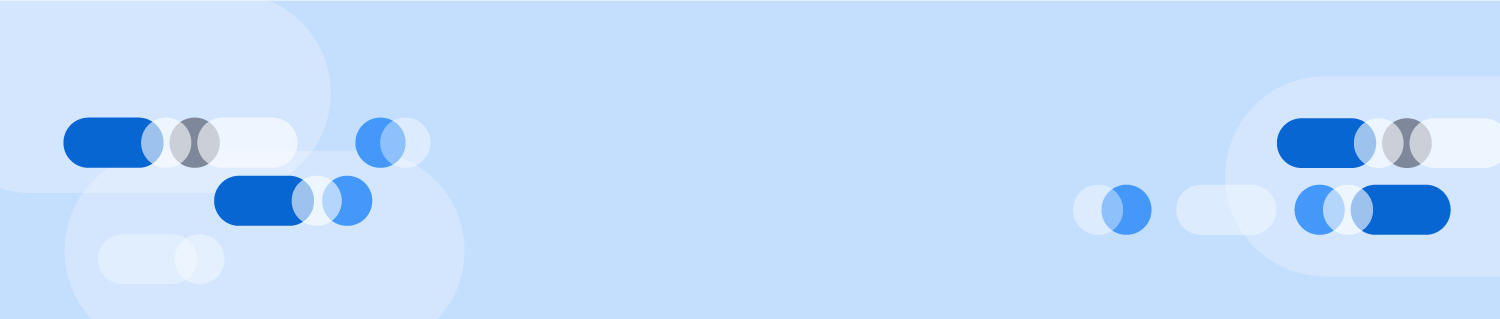
데이터 마이닝
데이터 마이닝의 정의
데이터 마이닝은 결과를 예측하기 위해 대량의 데이터 세트에서 이상점(anomalies)과 패턴 및 상관 관계를 찾아내는 프로세스입니다. 다양한 분석 기법을 통해 데이터를 활용하면 수익 증대, 비용 절감, 고객 관계 개선, 위험 감소 등의 효과를 경험할 수 있습니다.
데이터 마이닝의 역사와 현재 위상
데이터를 분석해서 숨은 연관성을 찾아내고 미래 동향을 예측하는 프로세스는 깊은 역사를 가지고 있습니다. "데이터베이스에서 지식 발견"이라고도 일컬어지는 "데이터 마이닝"이란 용어는 1990년대만 해도 존재하지 않았습니다. 그러나 그 근간은 통계(데이터 관계에 대한 수치 연구),인공 지능(인간을 모방한 소프트웨어 및/또는 기계의 지능), 그리고 머신 러닝(데이터를 통해 학습하고 예측하는 알고리즘)이라는 세 개의 긴밀한 과학 분야로 구성되어 있습니다. 데이터 마이닝 기술은 빅데이터의 무한한 잠재력과 경제적인 컴퓨팅 기능에 발맞춰 진화를 거듭하면서 새로운 기술로 거듭났습니다.
지난 10년간 데이터 처리 능력과 속도가 발전함에 따라, 지루하고 시간이 많이 소요되는 수작업에서 벗어나 쉽고 빠른 자동 데이터 분석을 활용할 수 있게 되었습니다. 수집한 데이터 세트가 복잡할수록 관련된 분석 정보를 찾아낼 가능성도 높아집니다. 소매, 은행, 제조, 통신, 보험업계의 경우 가격 최적화, 프로모션, 인구 통계부터 경제, 위험, 경쟁, 소셜 미디어가 비즈니스 모델과 수익, 운영 및 고객 관계에 미치는 영향에 이르기까지 모든 관련성을 파악하는 데 데이터 마이닝을 사용하고 있습니다.
Why is data mining important?
So why is data mining important? You’ve seen the staggering numbers – the volume of data produced is doubling every two years. Unstructured data alone makes up 90 percent of the digital universe. But more information does not necessarily mean more knowledge.
Data mining allows you to:
- Sift through all the chaotic and repetitive noise in your data.
- Understand what is relevant and then make good use of that information to assess likely outcomes.
- Accelerate the pace of making informed decisions.
- Uncover new insights from data through the use of predictive analytics.
데이터 마이닝이 중요한 이유
그렇다면 데이터 마이닝은 왜 중요할까요? 믿기 어렵겠지만 데이터량은 2년마다 두 배씩 증가하고 있습니다. 비정형 데이터가 디지털 세계에서 차지하는 비중은 무려 90%에 달하고 있습니다. 그러나 정보가 많아진다고 해서 반드시 인사이트가 늘어나는 것은 아닙니다.
데이터 마이닝은 다음과 같은 기능을 제공합니다.
- • 무질서하고 불필요한 데이터를 모두 사전에 걸러낼 수 있습니다.
- • 관련성 있는 정보를 파악한 후 그 정보를 십분 활용하여 경우의 수를 예측할 수 있습니다.
- • 정보를 바탕으로 의사 결정을 신속하게 내릴 수 있습니다.
데이터 마이닝 전격 해부, 데이터 마이닝 전격 해부에서 데이터 마이닝 기법에 대해 자세히 알아보세요. 이 백서에는 기업이 예측 분석과 데이터 마이닝 기법을 사용하여 데이터에서 새로운 분석 정보를 도출하는 방법이 소개되어 있습니다.
Data Mining in Today's World
Data mining is a cornerstone of analytics, helping you develop the models that can uncover connections within millions or billions of records. Learn how data mining is shaping the world we live in.
데이터 마이닝 소프트웨어
SAS의 데이터 마이닝 소프트웨어는 검증된 최첨단 알고리즘을 사용하여 가장 어려운 문제를 해결하도록 설계되었습니다.
사용 대상
데이터 마이닝은 다양한 산업과 분야에서 이뤄지는 분석 작업의 핵심입니다.
In an overloaded market where competition is tight, the answers are often within your consumer data. Telecom, media and technology companies can use analytic models to make sense of mountains of customers data, helping them predict customer behavior and offer highly targeted and relevant campaigns.
통신
경쟁이 치열한 통신 시장에서 살아남기 위해서는 소비자 데이터 안에서 그 답을 찾을 수 있습니다. 멀티미디어 및 통신 회사는 분석 모델을 이용해 수많은 고객 데이터를 파악하여 고객 행동을 예측하고 최적화된 타겟 프로모션을 실시할 수 있습니다.
With analytic know-how, insurance companies can solve complex problems concerning fraud, compliance, risk management and customer attrition. Companies have used data mining techniques to price products more effectively across business lines and find new ways to offer competitive products to their existing customer base.
With unified, data-driven views of student progress, educators can predict student performance before they set foot in the classroom – and develop intervention strategies to keep them on course. Data mining helps educators access student data, predict achievement levels and pinpoint students or groups of students in need of extra attention.
보험
보험 회사는 분석 노하우를 바탕으로 사기, 규정 준수, 위험 관리 및 고객 이탈과 관련된 복잡한 문제를 해결할 수 있습니다. 보험 회사는 데이터 마이닝 기술을 이용해 여러 비즈니스 부문에서 상품 가격을 보다 효과적으로 책정하고, 기존 고객 기반에 경쟁력 있는 상품을 제공하는 새로운 방법을 찾아냅니다.
Aligning supply plans with demand forecasts is essential, as is early detection of problems, quality assurance and investment in brand equity. Manufacturers can predict wear of production assets and anticipate maintenance, which can maximize uptime and keep the production line on schedule.
교육
교육자는 데이터를 토대로 학생의 학업 진도를 파악하여 강의실에 들어서기도 전에 학생의 성적을 예측하고 학생이 강의를 따라오는 데 도움이 될만한 대처 전략을 개발할 수 있습니다. 데이터 마이닝은 교육자가 학생 데이터를 토대로 학업 성취도를 예측하고 각별한 관심을 요하는 개인이나 집단을 정확히 찾아내는 데 유용합니다.
Automated algorithms help banks understand their customer base as well as the billions of transactions at the heart of the financial system. Data mining helps financial services companies get a better view of market risks, quickly detect and prevent fraud, manage regulatory compliance obligations and get optimal returns on their marketing investments.
Large customer databases hold hidden customer insight that can help you improve relationships, optimize marketing campaigns and forecast sales. Through more accurate data models and marketing analytics, retail companies can offer more targeted campaigns – and find the offer that makes the biggest impact on the customer.
제조
문제 조기 발견, 품질 보증, 브랜드 가치에 대한 투자와 마찬가지로, 공급 계획을 예상 수요에 맞추는 것 또한 중요합니다. 제조업체는 생산 설비의 수명을 예측하고 유지 보수를 예상하여 가동 시간을 극대화하고 생산 라인을 일정대로 유지할 수 있습니다.
금융
자동화된 알고리즘은 은행이 금융 시스템의 핵심인 수십억 건의 거래와 고객 기반을 이해하는 데 유용합니다. 데이터 마이닝은 금융 서비스 회사가 시장 위험을 보다 정확히 파악하고, 사기를 빠르게 감지하며, 규제 준수 의무를 관리하고, 마케팅 투자 효과를 극대화하는데 도움이 됩니다.
작동 원리
복합 분야인 데이터 마이닝은 다양한 분석 기술에 사용되는 다양한 방법 내지 기술로서, 기업의 전반적인 요구를 해결하고 다양한 유형의 질문을 제기하며, 다양한 수준의 인적 지식이나 규칙을 이용해 결론에 도달하는 것을 목적으로 합니다.
서술적 모델링(Descriptive Modeling): 서술적 모델링은 성공이나 실패의 원인을 규명하기 위해 기록 데이터에서 공통된 유사성을 찾아내는 데 사용됩니다(예: 제품 선호도나 정서에 따라 고객을 분류). 대표적인 기법은 다음과 같습니다.
클러스터링 (Clustering) | 유사 레코드 분류 |
이상 감지 (Anomaly detection)
| 다차원 이상치 식별
|
연관 규칙 학습 (Association rule learning)
| 레코드 간 관계 탐지
|
주요 구성요소 분석 (Principal component analysis)
| 변수 간 관계 탐지 |
관련성 분류 (Affinity grouping) | 공통 관심사나 유사한 목표를 가진 사람들(예: X를 구매하는 사람들은 종종 Y와 Z도 구매합니다)을 하나의 그룹으로 분류합니다. |
예측 모델링(Predictive Modeling): 이 모델링은 미래의 이벤트를 분류하거나 알 수 없는 결과를 추정하는 데 주로 사용됩니다(예: 신용 점수를 토대로 개인의 대출 상환 가능성을 파악). 예측 모델링을 통해 고객 이탈, 마케팅 반응 또는 채무 불이행 등에 대한 분석 정보를 얻을 수 있습니다. 대표적인 기법은 다음과 같습니다.
회귀분석 (Regression) | 하나의 종속 변수와 일련의 독립 변수 사이의 관련성 정도를 측정 |
신경망 (Neural networks) | 패턴을 감지하고 예측하며 학습하는 컴퓨터 프로그램 |
의사 결정 트리 (Decision trees) | 모든 발생 가능성을 각기 하나의 가지로 표현한 트리 형태의 다이어그램 |
서포트 벡터 머신 (Support vector machines) | 관련 학습 알고리즘이 사용되는 지도 학습 모델 |
처방적 모델링(Prescriptive Modeling): 웹, 메모 필드, 서적, 이메일, PDF, 오디오 및 기타 텍스트 소스에서 비정형 데이터가 증가함에 따라, 데이터 마이닝 관련 분야로 텍스트 마이닝을 도입하는 사례도 크게 증가했습니다. 예측 정확도를 높이기 위해 예측 모델에 비정형 데이터를 추가하려면 비정형 데이터의 구문 분석과 필터링 및 변환 작업을 수행할 수 있어야 합니다.
결국, 전처리(데이터 준비, 데이터 탐색)와 후처리(모델 검증, 스코어링, 모델 성능 모니터링)가 지극히 중요하므로 데이터 마이닝을 별개의 독립적인 개체로 생각해서는 안 됩니다. 처방적 모델링은 내부 및 외부 변수와 제약 조건을 검토하여 하나 이상의 대처 방안(예: 각 고객에게 보낼 최선의 마케팅 상품 결정)을 제시합니다. 대표적인 기법은 다음과 같습니다.
예측 분석 및 규칙(Predictive analytics plus rules) | 패턴을 토대로 if/then 규칙을 수립하여 결과를 예측 |
마케팅 최적화(Marketing optimization) | ROI를 극대화하기에 가장 유리한 미디어 혼용 전략을 실시간으로 시뮬레이션 |
이 주제에 관한 더 많은 글 읽기
-
Analytics: A must-have tool for leading the fight on prescription and illicit drug addictionStates and MFCUs now have the analytics tools they need to change the trajectory of the opioid crisis by analyzing data and predicting trouble spots – whether in patients, prescribers, distributors or manufacturers. The OIG Toolkit with free SAS® programming code makes that possible.
-
Machine learning for beginners and beyondWhether you’re an experienced data scientist or a machine learning beginner, you’ll appreciate these 10 tips for getting started with machine learning.
-
5 steps to sustainable GDPR complianceFollow these steps to achieve GDPR compliance by the May 2018 deadline – and get added benefits along the way.
-
Exploring the sun with big dataResearchers working for NASA are using automatic, exploratory and visual analysis of big data to help understand the mysteries of our universe.