Industrialize your analytics today
Learn how an analytics factory can be a conveyor belt for your data
By Adrian Jones, SAS
How can you incorporate more data into your systems, spread the use of predictive analytics throughout your organization and ensure the quality of reporting in every department?
Picture your data on a factory assembly line. As you collect it, data flows through an inventory or data preparation system that sorts and classifies the data based on how it will be used. Next, the data moves into production and packaging, where it is used for model building and analysis. The final step is distribution or deployment, where reports and results are delivered.
We like to think of this as an analytics factory, which creates an interesting metaphor for industrializing the analytical process. Let’s explore each step in more detail, paying attention to the benefits you realize with a factory for your analytics.
- Data preparation
The data preparation phase is about getting data to the analysts in a structured, timely manner. There are many ways to acquire data, from copying a single data set to receiving production data from IT. The analytics factory provides an approach in the middle that offers flexibility for analysts and a structured framework for governance. It makes sense for a lightweight process to support a one-off activity during model build. However, when the data needs to be accessed by many users in production processes, you need to make sure appropriate performance, measures and controls are in place.
The analytics factory is all about defining a set of processes that will
support analytics. It requires participation from many different parts
of the organization and requires the commitment of senior
managers to drive any such cross-functional alignment
.
Your data preparation process should make it easy to quickly move between the two states. This step requires the creativity of the business combined with the process and operational efficiency of IT. Taking a factory approach to data preparation will remove this gray area from many organizations, reducing the conflict that comes through duplications and inefficiencies.
- Model development
The model development phase should ensure that the analysts have a flexible high-performance environment with access to the data that they need. The analytics factory provides a well-managed central environment so users can focus on the task at hand rather than worrying about where to do the processing.
- Model management
Closely linked to model development is model management, which – in the factory setting – provides modeling workflow with documentation, notes, code and data samples inside a change log. This is important when working in an ever-more-regulated world. The factory approach also makes sure that appropriate governance is applied when working with larger data and processing resources in the organization. Likewise, appropriate approval and controls demonstrate that the model as an asset is ready for consumption within the organization.
- Model deployment
Model deployment links together the data preparation, model development and model management steps. In the analytics factory, model deployment will take outputs from each previous stage to create a production model package that can be deployed into an operational process. In the analytics factory, this step focuses on operational efficiency and should be executed in a controlled manner.
Modern developments in data platforms and analytical processing offer many technology options for improving the performance of the scoring or analytical processing. The analytics factory approach will make sure none of the business logic is lost when the model is deployed on a different platform. The result? Analysts have more confidence in the model, and the IT teams have the ability to deploy the model in the most efficient manner. Using the factory approach, this step should help build trust between the business and IT teams.
- Operational systems
Operational systems are the start and end of the process. The data is ultimately sourced from your operational systems in the beginning of the process, and then the analytical output is consumed by an operational process or application in the end. These systems are tightly controlled due to the operational dependency placed on them. Therefore, any interactions with them need to follow strict operating rules.
A factory approach defines appropriate interfaces and standards to ensure the data is available downstream and that any analytics deployed will be in a production state. These standards are managed closely by the operational teams with the handoffs well-defined in advanced. As you can see, the analytics factory is all about defining a set of processes that will support analytics. It requires participation from many different parts of the organization and requires the commitment of senior managers to drive any such cross-functional alignment.
Multiple benefits from the factory floor
Understanding how to deliver analytics in both tactical and strategic modes is vital for your analytics factory to work. Many organizations struggle to make progress because analytical projects are disconnected from the other systems and processes within the organization.
With the analytics factory, you can make those connections and industrialize the analytics life cycle. You’ll also be bringing efficiency and value to the process by scaling out the production of analytics.
To employ some of these concepts, you must become more agile in the way you use and deploy analytics. But you will drive more efficiencies and avoid unnecessary technology upgrade costs. Ultimately, if you can further the use of analytics in your organization, you will see widespread benefits from the improved decisions throughout the organization and the time savings for analysts, IT and decision makers.
Adrian Jones is a member of the SAS Analytical Platform Centre of Excellence, where he focuses on high-performance analytics and enterprise architecture. He helps organizations use data and analytics strategically by optimizing their data and analytics architectures. This role involves new product direction as well as work with customers to apply lessons in the field.
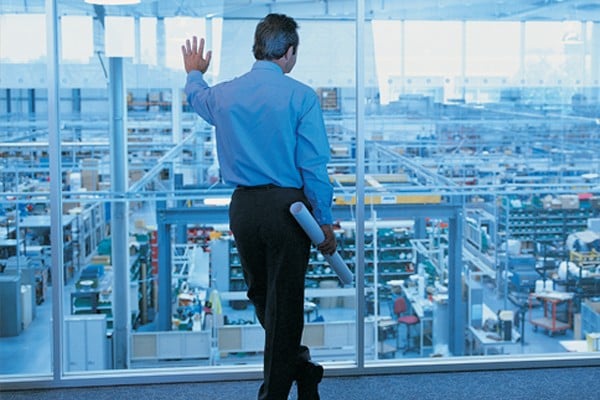
Read More
- Return to Analytics Insights.