Data analytics for utilities in Australia: What, why and how
How can big data analytics help Australia's energy utilities?
In this article, we discuss why you should care about data, and how it can improve your service
In the Australian energy utilities sector, you've likely heard that some of your competitors are investing in the science of data - putting great stake in the internet of things (IoT) and business intelligence tools to gain detailed customer insights and get a leg up on the competition. Many see big data analytics as the future of the industry, but what does it mean for utilities in Australia, and how can they use it?
What could data analytics mean for Australian utilities?
Companies around the world are gathering a host of information - sensor and network data, customer attitudes and demographics, billing histories, energy demand forecasts, and more - in an effort to create a more personalised, flexible service.
Customer satisfaction is the next major battleground for Australian utilities.
Customer satisfaction, according to EY, is the next major battleground for Australian utilities. The company's Digital Australia report suggests that Australia is lagging behind the rest of the world when it comes to innovation and customer happiness.
But yet, the solutions are already close at hand. EY goes on to recommend that local utilities adopt analytics in order to provide a "deeper, more intuitive service."
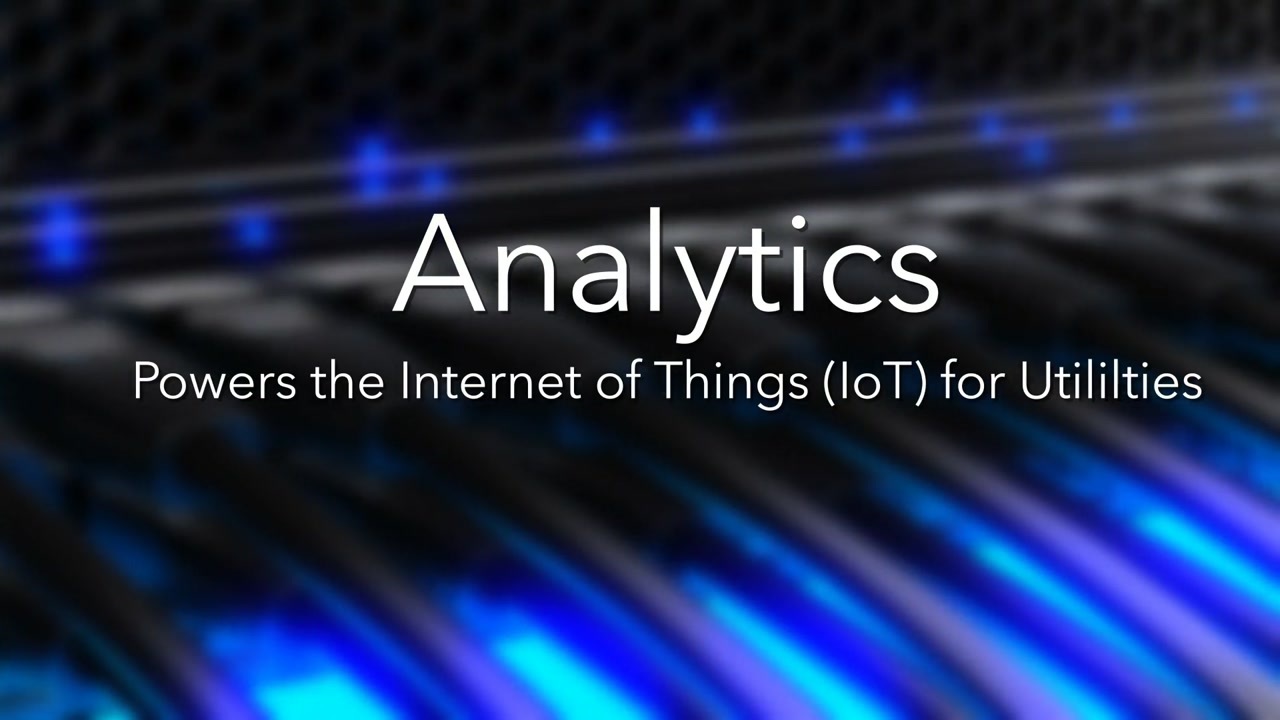
- 2x
- 1.75x
- 1.5x
- 1.25x
- 1x, selected
- 0.75x
- 0.5x
- Chapters
- descriptions off, selected
- subtitles settings, opens subtitles settings dialog
- subtitles off, selected
- English Captions
- Japanese Captions
This is a modal window.
Beginning of dialog window. Escape will cancel and close the window.
End of dialog window.
This is a modal window. This modal can be closed by pressing the Escape key or activating the close button.
This is a modal window. This modal can be closed by pressing the Escape key or activating the close button.
This is a modal window. This modal can be closed by pressing the Escape key or activating the close button.
This is a modal window. This modal can be closed by pressing the Escape key or activating the close button.
Utility analytics in 2017: Aligning data and analytics with business strategy
Download the Utility analytics in 2017 whitepaper, which explores the issues and trends that are shaping how utilities are deploying data and analytics to achieve their business goals.
Free utility analytics whitepaper
Consider analytics in the context of weather disasters
The modern world is not as simple for energy utilities as it used to be. The simple addition of renewable energy like wind and solar means the grid is less stable, and more prone to sporadic events. But this is one instance where the internet of things can be linked with powerful analytics tools to keep companies one step ahead of chance.
For example, imagine collecting all of the data from your company's PMU sensors and storing them using a cost-effective Hadoop framework - even though we're talking about a near-uncountable amount of data, it can be easily stored, accessed and, more importantly, analysed.
One day, a weather event strikes a large population of your customers and the grid goes down, or is damaged in some capacity. With the right data, you can quickly diagnose the error - was it equipment failure, a lightning strike, or something new? - and assess the impact at, fittingly, lightning speed. Your technicians can go out to repair the damage, while your customer service agents answer the phones armed with the right knowledge to assure customers that their power will return soon.
With the power of storing and analysing data, you've created a better customer experience. And this data can be further analysed to predict these events in the future, making you more efficient at dealing with them every time.
Examples of how utilities are using big data analytics
Let's examine two case studies of utilities companies in Australia and around the world utilising big data analytics to improve their service.
Forecasting future energy demand in an increasingly renewable
energy world isn't exactly easy.
At home in Australia
Take Origin Energy, for example. When the Origin team started talking to us here at SAS, they wanted to better understand their customers' demands. But of course, as we know, forecasting future energy demand in an increasingly renewable energy world (and a country with unstable weather like Australia) isn't exactly easy.
Well, this is where we deployed SAS Energy Forecasting. By using Origin's collected data and SAS's powerful tool, the company could move away from the traditional monthly bill of random pricing to a Predictable Plan of charging customers the same amount each time. Now customers can budget confidently, and are never shocked by an unexpectedly high energy bill. This has been highly successful in increasing Origin's customer loyalty.
An overseas example
What if your company experiences large amounts of churn? Collecting data and analysing it closely can help here too, just like it has helped EDF Energy in the UK.
EDF collects three data sets to try and classify customers and identify who is most likely to leave. By comparing attitudes with lifestyles and demographics, EDF - through the power of SAS analytics - can help its marketing team prioritise customer communications based on identified risk. For example, EDF salespeople can focus on upselling customers who are actually likely to buy, instead of rocking the boat with those who are not.
Now although these two examples only scratch the surface of how big data analytics can help utilities companies in Australia, you begin to see that nearly any customer or service problem can be solved with the right information at your fingertips. If you'd like to learn more about how SAS can help your company, get in touch with our team today.
Recommended reading
-
Article A data scientist’s views on data literacyData literacy is a social imperative – and understanding data and data analysis is critical for being a responsible citizen. Get a data scientist and teacher's perspective on the value of having foundational knowledge so you can more easily tell data facts from data fiction.
-
Article Understanding capital requirements in light of Basel IVMany financial firms are already using a popular 2012 PIT-ness methodology for internal ratings-based models. This article examines eight ways the industry is successfully using the methodology – and why this approach can bring synergies for banks, value for regulators, and major competitive advantages.
-
Article Edge computingWith edge computing, IoT data is processed at the edge of a network where it’s created or collected – avoiding delays and enabling real-time processing and action.
-
Article How to drill a better hole with analyticsFrom drilling holes to preventing health care fraud, learn about some of the new technologies SAS has patented with IoT and machine learning technologies.