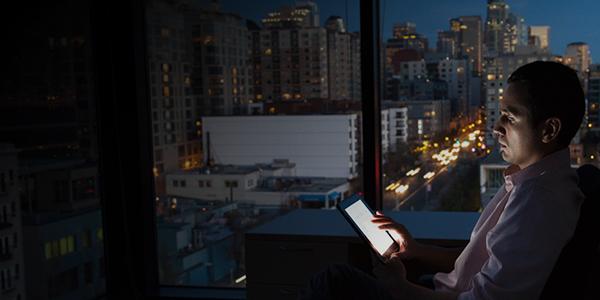
4 strategies that will change your approach to fraud detection
Fraudsters and money launderers are getting more sophisticated with their tactics. We’re getting more sophisticated at fighting back.
Thomas French, Senior Financial Industry Consultant, SAS Security Intelligence Practice
Eric Herson, Principal Industry Consultant, SAS Security Intelligence Practice
Financial institutions are doing business in a far riskier world than before. According to The Identity Theft Resource Center in Q3 2022, the numbers are sobering:
- Fraudulent activity is growing. More than 105 million victims were affected by data compromises in Q3 2022, a 72% increase over the first half of the year. The number of data compromises reported in Q3 2022 (474) reflected an increase of more than 14% over Q2 of the same year and more than 17% over Q1 2022.
- Fighting fraud is becoming more costly. The 2022 Anti-Fraud Technology Benchmarking Report from ACFE (sponsored by SAS) shows that most organizations are already budgeting for expanded technology use to fight fraud. Respondents indicated that 60% expected a significant (17%) or slight (43%) budget increase for anti-fraud technology in the next two years.
- The top obstacle to enacting a new anti-fraud technology is budget (and related financial restrictions), according to the ACFE study. In fact, 78% of participants said this is a major or moderate challenge at their organization. Adopting new technology in an organization can come with challenges both in the planning and implementation stages.
Fine-tune your fraud detection strategy
“Fraud doesn’t go away, it’s always changing … and fraud executives must continually invest in anti-fraud technology that goes beyond a single-point solution,” says Thomas French, industry advisor for fraud at SAS. “A business case just won’t deliver a strong enough ROI to warrant the outlay. They have to look deeper to consider (and account for) the impact of fraud on the consumer.”
In their efforts to improve the customer experience while maintaining a robust fraud detection strategy, organizations must use and manage multiple sources of data (both structured and unstructured). Anti-fraud data analytics initiatives typically include internal structured and unstructured data, public records, law enforcement or government watchlists, social media, other third-party data and data from various types of connected devices.
The good news is that advances in fraud prevention and detection technologies give financial institutions more accurate and efficient solutions than ever for uncovering the risk of fraud and financial crimes. Here are four ways to improve your organization’s risk posture.
Global digital fraud trends report
Criminals continually find new ways to exploit digital channels. Javelin evaluated digital fraud trends and implications across 12 countries, making eight broad recommendations for combating the issue. Their report also explains why machine learning and biometrics are so crucial to successfully combating digital fraud.
1. Add AI and machine learning to improve fraud detection accuracy and efficiency
It’s proven that machine learning (ML) helps organizations find more fraud faster and earlier. A form of artificial intelligence (AI), ML is powerful for improving both the accuracy and efficiency of fraud detection and prevention strategies in a real-time environment. Applying machine learning to continuously monitor for fraud is a tried-and-true approach to fighting financial crimes.
- Supervised machine learning algorithms can self-learn from target variables within the data, flag anything that doesn’t fit the norm, then apply this knowledge to new and unseen data.
- Unsupervised machine learning uncovers potentially suspicious types of risks you might not think to look for. It works without being given a target. Instead, it searches for anomalies in the data.
Combined in an ensemble model, machine learning provides a wide breadth of coverage across current risks, as well as new and emerging threats. On top of that, ML reduces false positives while identifying previously unknown risks.
For example, SAS deployed a digital payment model that demonstrated rapid success in real-time fraud detection. It detected 50% of fraud – alerting on just 0.5% of the portfolio, with very few false transactions.
With machine learning, anti-fraud systems can automatically:
- Suggest and update rules for detection and alerts. ML examines large amounts of data to help establish rules and keep them current.
- Select the most accurate detection models. Applying a combination of machine learning techniques delivers the most accurate detection rates. This approach for detecting and preventing fraud allows newer techniques, like gradient boosting and support vector machines, to enhance proven methods like neural networks.
- Automate processes for investigation. On average, 60% to 70% of an investigator’s time is spent collecting data about a subject to detect fraud. Machine learning can guide systems to automatically search and retrieve data, run database queries and collect information from third-party data providers without human intervention. SAS has helped clients reduce time to case decision by 20% to 30%.
Unlike rules, which are easy for fraudsters to test and circumvent, the application of machine learning through analytics has been the standard for the SAS® fraud and financial crimes solutions for many decades.Jim Goodnight CEO SAS
2. Coordinate and operationalize fraud, anti-money laundering (AML) and cyber events
Financial institutions are taking advantage of big data analytics to consolidate data across typically siloed functions. Today, it makes sense to bring these functions together for a more holistic view of risk. Much of the data is similar, and regulations are pushing risk identification to real-time. There’s also an opportunity to reduce operational costs and enhance efficiency while developing a cross-functional view.
For example, whether reviewing a loan application, making a decision about a payment transaction or uncovering terrorist financing through anti-money laundering efforts, there are opportunities to learn from every interaction. Achieving real-time agility in a world of faster payments is critical because retail and financial entities must adapt to improve customer experience amid demands for convenience. Such efforts, however, intensify the risk environment.
- Mobile and online commerce reshape expectations for speedy authentication across all contact channels. Financial institutions need a combination of customer, device and session behavioral analyses to reduce fraud and prevent losses.
- More forward-thinking intelligence units are finding common elements – domain names, IP addresses, devices, etc. – that reveal criminal organizations previously undiscovered by siloed functions.
- The demand for real-time funds availability began with the UK banking initiative Faster Payments Service (FPS), which reduces payment clearing times from three working days under the legacy BACS system to a few hours. New payment systems in the US and Australia – coupled with the growth of fintechs – are bringing this down to a few seconds.
Once a luxury, real-time transaction monitoring is now a baseline requirement for all payment types. To counter payment fraud, it’s vital to incorporate not only financial transactions but also authentication, session, location and device event data.
3. Streamline know your customer (KYC) processes
High-profile leaks such as the Panama Papers dramatize the need for more transparency into the real owners or beneficiaries of corporate and legal entities. At the same time, the financial industry has seen deposit account and credit application fraud rise to 20% of all banking fraud. Both realities redefine the expectations for KYC processes. SAS can help by:
- Fortifying and speeding authentication processes that validate digital devices and in-person applicants.
- Using robotic process automation (RPA) to automate searches and queries of third-party data during enhanced due diligence processes.
- Supporting new data elements, such as ownership percentages and controlling interests.
- Offering investigative interfaces that streamline the ad hoc process of gathering external unstructured information, including numbers, text, images and video.
For document query and retrieval, image recognition capabilities based on natural language processing techniques have shown remarkable results. In one test case, SAS reduced the time to identify, classify and analyze trade documents from 700 hours to a matter of minutes.
In another pilot, we scanned approximately 9,000 SWIFT messages looking at Palestinian boycott language. A human would need about five to seven minutes to review each message. During this pilot, we found we could do image recognition and contextual analysis of those messages in less than one second per message.
4. Improve investigation efficiency with intelligent case management.
Much of the initial adoption of AI focuses on automating manual processes to reduce the costs of running an effective fraud and financial crimes investigation. Investigators shouldn’t spend their valuable time on rote tasks that machines can do better. An advanced analytics-driven alert and case management solution that presents a single view of data can automatically:
- Prioritize cases, recommend investigative steps and fast-track straightforward cases.
- Enrich alerts with details about the associated customers, accounts or beneficiaries.
- Intelligently find and pull data for a case from internal databases or third-party data providers.
- Present data in easy-to-understand visualizations on one screen.
- Auto-populate and prepare suspicious activity alerts (SARs) for electronic filing if applicable.
Why SAS?
An adaptive learning system from SAS combines embedded analytics with alert and case management capabilities to automate processes and continuously learn from outcomes. Through alert prioritization and triage, investigative processes and dashboards, this approach adapts continuously to new financial crimes risks.
About the Authors
Thomas French, Senior Financial Industry Consultant, SAS Security Intelligence Practice
Thomas French is a strategic leader with 28 years of experience in consumer and commercial bank fraud prevention, detection, customer service, claims and recoveries. He works with financial services companies across the globe using SAS to combat bank fraud, AML, cyber threats and internal fraud – and to mitigate operational and reputational risk.
Eric Herson, Principal Industry Consultant, SAS Security Intelligence Practice
Eric Herson is a liaison between SAS’ global client-facing account teams and financial crimes product development and delivery organizations. He has more than 40 years of experience assisting clients globally with complex data-driven fraud and analytics projects. Prior to joining SAS in 2010 to concentrate on real-time fraud and AML/regulatory initiatives, he worked for both FICO and First Data International in sales management and divisional P&L leadership roles.
Recommended reading
-
Article Analytics: A must-have tool for leading the fight on prescription and illicit drug addictionStates and MFCUs now have the analytics tools they need to change the trajectory of the opioid crisis by analyzing data and predicting trouble spots – whether in patients, prescribers, distributors or manufacturers. The OIG Toolkit with free SAS® programming code makes that possible.
-
Article When it matters: Safeguarding your organization from the insideWith evolving threats, fraud detection technologies have to be flexible and nimble, and automated risk detection is a crucial component of decision advantage.
-
Article How to uncover common point of purchaseBanks that want to stay ahead of CPP and contain the costs of fraud need to implement advanced anti-fraud techniques.
-
Article Exploring the sun with big dataResearchers working for NASA are using automatic, exploratory and visual analysis of big data to help understand the mysteries of our universe.
Ready to subscribe to Insights now?