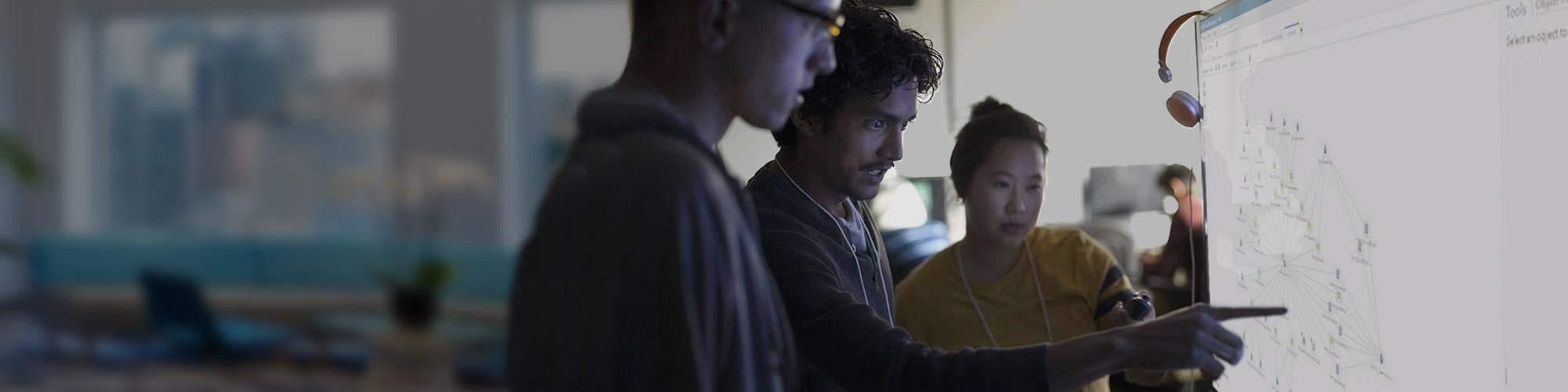
Finding the Fraudsters Within
How analytics can ferret out rogue insurance professionals who game the system.
Amanda Holden, National Executive Fraud & Security Intelligence SAS Canada
We all know the insurance industry is under siege by fraudulent activity from the outside. Examples abound of unscrupulous actors — including rehab and health care providers, car repair facilities, tow truck drivers, lawyers and claimants — seeking to game the system. Last year, calling auto insurance fraud a systemic “industry” that costs its taxpayers $1.6 billion annually, the Ontario government vowed to create a Serious Fraud Office to tackle the problem.
But what about those attacking the system from within?
Last year, a partner in an insurance firm based in Lethbridge, Alta. pled guilty to a charge of bilking the company out of half a million dollars in commissions over the last decade, by creating fake accounts and altering existing ones. The alleged fraud only came to light after another employee reported anomalous activities in the partner’s book.
One personal and commercial insurer estimated that internal fraud might amount to as much as 10 per cent of premium volume.
The techniques of fraudsters within the P&C industry are varied. Among the most common are:
- skimming or lapping premiums (funneling customer premiums into fake accounts);
- fraudsters use their own money to create fake policies to score commissions, incentives, and bonuses, and then let the policies lapse;
- forging policyholders’ signatures; and
- churning (convincing customers to terminate their policies and buy new ones, collecting extra commission in the process).
In some instances, agents may potentially write policies for higher-risk customers at a reduced rate (rather than one that might make the customer walk away), thereby collecting commission on policies that expose the company to higher payouts and inaccurate risk management.
One challenge for insurers lies in the velocity, variety and volume of networked transactions, which increase the threat exposure. Another is using limited investigative or audit resources effectively. How do you find a needle when you’re not even sure in which haystack its buried?
Fortunately, fraudulent activity leaves a data footprint that rings alarm bells in the analytical organization.
Simplifying Fraud Analytics
10 Steps to Detect and Prevent Insurance Fraud
DATA FOOTPRINT
The simplest definition of analytics is the process of turning data into insight. Sifting algorithmically through data, applying statistical principles, pattern recognition, data modelling, machine learning and other techniques, an analytics regimen can discover previously unknown connections that can predict outcomes, trace the symptoms of anomalies and help define more effective and efficient business rules.
If the sheer volume of data collection were the solution, we would be well on our way to a fraud-free world. The volume can be as much a liability as an asset, both in its sheer crushing weight and its bewildering variety: reports and spreadsheets, databases and time series, social media posts and email messages. It takes powerful technology — and more importantly, powerfully attuned business and data sense — to filter that ocean into a drinkable glass of water.
HAYSTACK INTO HAY BALE
There is a rich and deep catalogue of analytical techniques with a track record of preventing and predicting fraudulent activity. First steps need not be complex: good data, strong knowledge of business processes and simple business rules often find the low-hanging fruit. From there, an organization’s fraud analytics practice can grow in sophistication, balancing risk management and customer experience. Among those tools:
Peer grouping with geospatial and other agency attributes
Like-size agencies in similar geographies and demographics should have similar books. An agency that stands out for policies of particular advantage — for example, in professions that offer significant discounts or policy types that can reap better commissions and bonuses —merits further investigation.
Anomaly detection
Agencies that, for example, write a substantial amount of business outside their geographical area could be systematically flipping policies to increase commissions. In this example, fraud may be detected through “unsupervised learning” by an analytics system (the application of statistical techniques and analysis). There is also a category of “supervised learning,” which is taught through industry experience and a library of analytical precedent.
Target definition
Three years of policy, quote and claims data, together with a history of agent gaming, is typically enough for an analytical system to use machine learning to create accurate predictive models. Social network analysis Analytics systems can discover unexplained relationships of policy activity and entity — for example, apparently unrelated policies linked to a common telephone number or email address. Integrating these techniques into an overall analytics strategy can help flag gaming activity more often and more accurately, allowing investigative resources to be used in a more cost-effective manner.
ANALYTICS IN ACTION
The risk management practice of one of the largest insurers in the United States faced a challenge policing its huge number of agents and policies. Collating the documentation for a single case took 17.5 hours and still returned a false positive rate of nearly 50 per cent.
The company’s strategy to develop an analytics-based approach to the problem was threefold:
- Integrating policy, quote, claim and internal audit data, and then cleansing and standardizing it.
- Applying anomaly detection, predictive modeling and social network analysis to create an alert generation process.
- Creating a hybrid scoring system based on a combination of business rules and model and Social Network Analysis scores.
The result? Agent review time plunged from 17.5 hours to three. Having five times as much investigative time came in handy: the improved alert system flagged 10 times as many files.
BONUS POINTS
Fraud detection isn’t the only area that can reap efficiencies from an analytics strategy. The side effects of an anti-fraud initiative can lead to less flashy improvements such as closing system loopholes, flagging poor business practices, enabling automated reporting and monitoring, and aligning compensation practices. All of that is reflected in the bottom line. All these efficiencies are predicated on the collection and integration of data from across the organization. Data can overwhelm, or it can be mastered to improve the business. Advanced analytics is the key.
Learn more about SAS’ detection and investigation solutions for insurance here: https://www.sas.com/en_us/software/detection-investigation-for-insurance.html
This article was originally published in Canadian Underwriter Magazine
About the Author
Amanda Holden is a technology, fraud and operations leader with over 15 years’ experience in payments and 25-plus years’ experience in financial services. Amanda is focused on finding solutions to customers’ fraud, waste, loss and abuse problems. She is passionate about data and analytics and the role they play in reducing financial crimes and creating better public outcomes in Canada. Amanda works to bring business and technology together in partnership.
Recommended reading
-
What is synthetic data? And how can you use it to fuel AI breakthroughs?There's no shortage of data in today's world, but it can be difficult, slow and costly to access sufficient high-quality data that’s suitable for training AI models. Learn why synthetic data is so vital for data-hungry AI initiatives, how businesses can use it to unlock growth, and how it can help address ethical challenges.
-
6 ways big data analytics can improve insurance claims data processingWhy make analytics a part of your insurance claims data processing? Because adding analytics to the claims life cycle can deliver a measurable ROI.
-
Shut the front door on insurance application fraud!Fraudsters love the ease of plying their trade over digital channels. Smart insurance companies are using data from those channels (device fingerprint, IP address, geolocation, etc.) coupled with analytics and machine learning to detect insurance application fraud perpetrated by agents, customers and fraud rings.
Ready to subscribe to Insights now?
SAS® Viya™
Make analytics accessible to everyone and bridge the talent gap in your organization