Better Pipeline Forecasting through Analytics
By Ian Jones, Senior Strategist, Energy Risk Management
Reducing guesswork and man-hours are powerful incentives to embrace big data analytics in forecasting. That’s especially true when the forecast in question lays out capital planning for the year ahead, but takes most of the year to complete.
Take, for instance, the annual system design forecast produced by North American oil and gas pipeline operators. This annual forecast is both a regulatory requirement and a business necessity. It lays out for regulators, customers and other interested parties a company’s plans to build new pipeline and storage facilities. The forecast justifies this capital planning based on how much gas a pipeline will receive and deliver, where it expects to receive that gas and what markets are most likely to want it, the peak amount it anticipates moving through the pipeline and the ability of the pipeline system’s design to handle that capacity.
The forecast incorporates a range of economic assumptions, such as changes in the fundamentals of the energy market and the fluctuations of commodity prices. These are not small considerations: When hydraulic fracking enabled the New England region to source natural gas locally – and export some of it – their traditional suppliers in Canada, the American West and the Gulf Coast lost a customer and gained a competitor. The demand for long-haul pipeline heading east was reduced, and a scramble began to build enough local pipe in New England to handle the new capacity.
In another instance, demand for oil sands oil overseas led the Canadian National Energy Board to approve reversing the flow of Canada’s Line 9 pipeline so product could be moved from Alberta to ports on the East Coast, with plans to expand the pipeline to handle more capacity.
These are the kinds of market dynamics a pipeline company must incorporate into their forward view. Such significant shifts affect how the business operates and how they plan for the next two to five years in their annual system design forecast. But the forecast also requires looking inward, at data from wells, pools, meter stations and other sources. When more supply or demand than the existing pipeline can handle is forecast, new construction is proposed – if it’s feasible for the system to balance supply and demand on that route. Pipelines want to ensure their infrastructure is utilized as fully as possible, but a particular section of pipeline might be filled to capacity during days of peak flow and under-utilized on other days. Understand these usage patterns influences how a pipeline is operated and where new facilities or upgrades are required.
Not surprisingly, the forecasts used to make these expensive real-world decisions rely on an enormous amount of disparate data. Simply gathering the data is a complex and time-consuming process that draws from many distinct systems and sources, meaning additional man-hours must be devoted to integrating, cleansing and reconciling data. It also means precious hours that could be devoted to the more valuable task of analyzing the data are lost to managing it.
It’s here that an integrated data management and advanced analytics framework begins to make a difference. Automating the aggregation and processing of data, and preparing it for analysis, adds significant efficiency to routine data staging efforts and gets a forecaster to the analysis stage faster. When the majority of the forecasting process can be moved into data analysis, the quality and impact of that analysis naturally increases.
Once the data management piece is streamlined, advanced analytics steps in to take system design forecasting to the next level. Consider the decline curve analysis used to forecast supply in the convention oilfield. In the new world of shale production, the way yields from a well decline over time takes on different, more complex behaviors than traditional forecasting methods can capture accurately. Employing data-driven analytical processes in addition to decline curve analysis produces faster and more reliable forecasts.
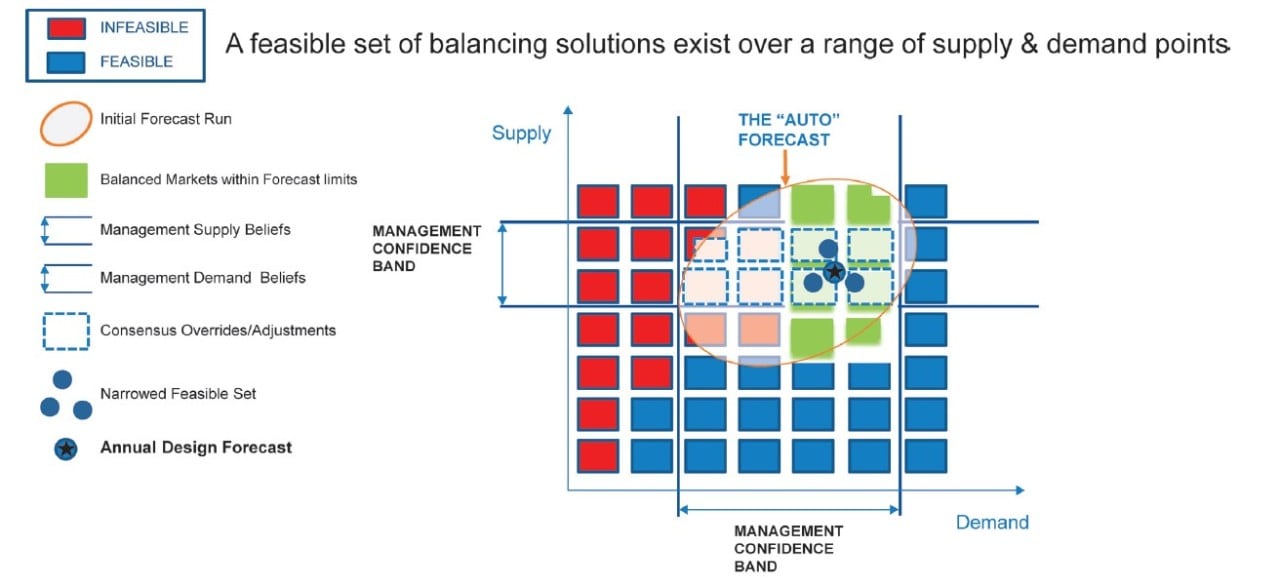
SAS has developed a seven-factor planning method, as illustrated above, that can increase the speed and accuracy of pipeline system design forecasts. An initial forecast based on supply capabilities and anticipated demand is run to determine a feasible set of balanced markets. Think of balancing as the process of matching natural gas supply and demand points to ensure sufficient pipeline capacity is available when delivery is scheduled. Many potential markets are not feasible because they lack the physical capacity to deliver the necessary volumes of gas from suppliers to customers; others don’t make economic sense.
This initial forecast can be optimized by applying analytics to that vast pool of aggregated data, in order to limit the outcome based on statistical confidence of probable supply and demand combinations.
The next crucial step is for a pipeline company’s management to scrutinize this initial forecast. They apply their experience, expertise and knowledge of their contractual obligations, in order to hone in on the potential balanced markets that would be most feasible. A consensus between the initial forecast and the markets that management thinks are most feasible leads to a smaller, more focused number of potential balanced markets.
Here advanced analytics comes into play again. Data-driven analytical models are capable of testing and refining the consensus forecast that weighs the initial statistical outcomes against management insights. This produces a much narrower set of feasible supply-demand combinations, which shows management where the most potentially profitable markets exist.
The result is a far more focused system design forecast than has been available without the aid of advanced analytics. As a result, management has a specific target for capital planning. They can confidently lay out their conclusions as to the best location for new and upgraded facilities, based on the most productive and economic paths from supplier to market.
For a deeper dive into this seven-factor planning method, download the new SAS whitepaper, Optimizing Pipeline System Design Forecasts: An Integrated Data Management and Advanced Analytics Approach.
Ian Jones is Senior Strategist for SAS’ energy risk management practice. Prior to joining SAS in 2009, he served as editor of the industry trade journal The Risk Desk.
Get More Insights
Want more Insights from SAS? Subscribe to our Insights newsletter. Or check back often to get more insights on the topics you care about, including analytics, big data, data management, marketing, and risk & fraud.